Quantum Algorithms for Machine-Learning Models, “Nature Communications“ paper by Jens Eisert et.al.
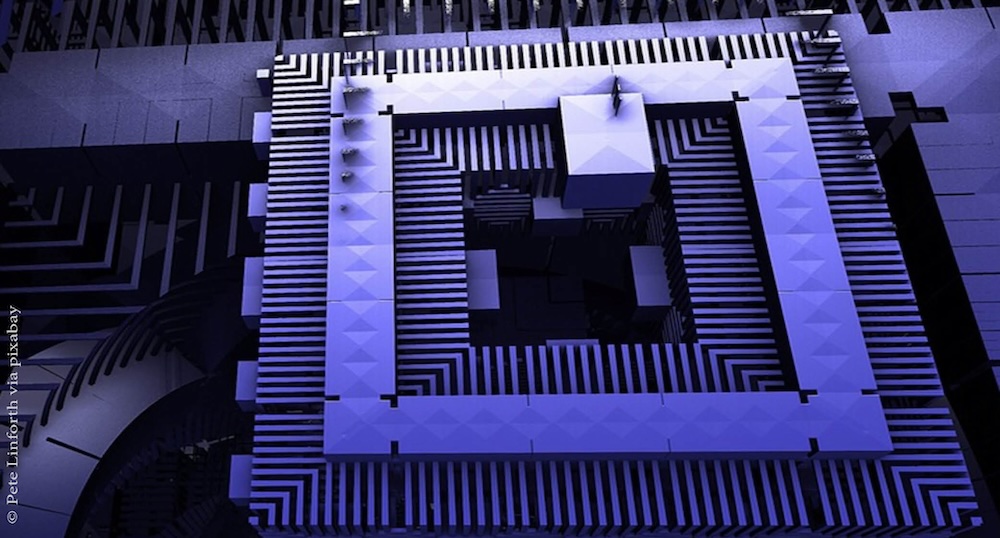
In the Nature Communications publication, an interdisciplinary team comprising mathematicians, computer scientists, and physicists from the University of Chicago, MIT, and FU Berlin investigated the potential of quantum computers in addressing machine learning problems. MATH+ member Jens Eisert contributed the central idea of how a quantum advantage could be created, originating from a MATH+ project.
Two promising fields: Machine Learning and Quantum Computing
Speaking to MATH+, Jens Eisert explains how this work intersects two intriguing and promising fields. Firstly, machine learning, which is rapidly transforming various domains. Any algorithm that makes predictions based on past data utilizes machine learning, as exemplified by the widespread usage of ChatGPT. Secondly, recent years have revealed the potential of quantum computing to solve certain structured issues efficiently. While many scientists are exploring this area, according to Jens Eisert, it remains a ‘hot’ topic with only heuristic explanations for its potential.
The concept of quantum computers originated with Feynman, Benioff, and Deutsch in the 1980s and has held significant theoretical promise since the 1990s. However, it’s only within the last five years that industry players have entered the arena, constructing physically large-scale quantum computers. These quantum computers we discussed have yet to materialize in their entirety. Still, five years ago, the currently existing devices were also considered science fiction. Thus, quantum technology still represents a form of future technology. Nonetheless, when finally fully realized, they possess the potential to solve specific problems exponentially faster and more efficiently than classical supercomputers, which is why there is an immense interest in quantum technology.
Quantum computers have the potential to train large-scale networks more effectively
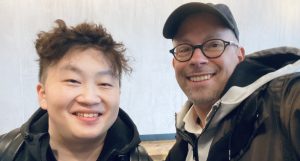
LIU Junyu (U Chicago) and Jens Eisert (FU Berlin)
The recently published paper in Nature Communications addresses the crucial question of how quantum computers could provide an edge in utilizing practical data that has thus far proven challenging for machine learning, such as generating images of dogs and cats. Generally, machine learning involves two key steps: training the networks using classical algorithmic methods and then applying them—namely, training and application. This process demands significant time and energy; for instance, Chat GPT alone required years of training. The team behind the publication has demonstrated in a super-polynomial separation that quantum computers possess the potential to train classical, large-scale networks more effectively, providing substantial computational benefits. This is a development that introduces an exciting new dimension. Eisert remarked, “The underlying architecture is not constituted by quantum networks, but rather about classical neural networks that must be adequately sparse.”
This approach could also be applied to significant practical datasets, including industrial datasets like those of the “CIFAR-100 dataset”(Krizhevsky, A., Nair, V. & Hinton, G. CIFAR-100, Canadian Institute for Advanced Research,2009). Classical computers were employed to quantitatively simulate this process to assess its practical viability. As a result, the practical question of whether quantum computers can more effectively train classical networks was explored using real-life industrial machine standards, combining applied mathematics with empirical evidence. This breakthrough garnered widespread recognition within the community, as the academic advantages were already acknowledged. Still, there needed to be more clarity on whether such methods could be practically implemented.
MATH+ projects utilize application-oriented mathematics to explore the advantages of quantum computers
Jens Eisert elaborates, “Within the framework of the MATH+ projects EF 1-7 and EF 1-11, along with Klaus-Robert Müller, our Berlin team is dedicated to exploring how quantum computers could provide advantages in machine-learning tasks. Up to now, this question has been tackled primarily using numerical trial and error methods. However, in a preliminary paper preceding this Nature Communications publication, we have theoretically demonstrated that quantum computers could solve certain constructed machine-learning problems exponentially faster than classical computers, presenting significant potential. This involves application-oriented mathematics with non-trivial methods and numerous theorems presented in the lengthy publication. Given the absence of equipment to execute the software, immediate application is not feasible.
Nevertheless, pragmatically driven individuals and leading players in the quantum industry are embracing these results. They seek precisely this type of application to propel the advancement of quantum computers, as many applications, while still theoretical, are recognized. Therefore, this work is regarded as a crucial step towards practical applications, aligning well with the goals of MATH+.”
Jens Eisert has even secured placement for two papers on machine learning in Nature Communications. The second is set to be published in the coming weeks. In the first paper presented here, which is co-authored with a colleague from the University of Chicago and in collaboration with MIT, Eisert is the sole contributor from his Berlin team. The second publication is a result of the work of his Berlin team. Both papers explore the intersection of machine learning and quantum computing, framing them within the context of MATH+ project EF 1-11.
Vast interest in developing quantum technologies and its application
The quantum community is vigorously working on both hardware and applications, with significant investments pouring into this field. In Germany, for instance, the government’s economic stimulus and future package allocates two billion euros specifically for developing quantum technology. With 500 employees dedicated to quantum topics, IBM emphasizes the critical importance of discovering practical applications. Jens Eisert asserts, “For those eager to innovate and build quantum computers, what we’re currently pursuing represents the most tangible application they’ve encountered in the past three years.”
It is challenging to predict the availability of quantum computers, with estimates ranging from years to decades. While significant progress has been made theoretically and mathematically over the past two decades, practical technological advancements in quantum device development have only been made in the last five years, resulting in devices with up to 1000 qubits. For comparison, at the beginning of Jens Eisert’s academic career, only one qubit was available. While this rapid development underscores the progress, it remains insufficient for achieving the desired quantum algorithm. Nonetheless, Eisert emphasizes that they are close, stating, “The important and difficult question is whether we can effectively address errors.” However, a recent breakthrough sparked optimism within the scientific community. Harvard-MIT’s QERA company surprised many by announcing a dataset demonstrating successful quantum error correction with 48 error corrected, error-protected quantum bits—a milestone initially expected to take decades to achieve.
Of course, the field of quantum computing is surrounded by considerable hype. High expectations, substantial investments, and extensive media coverage are driving forces. Eisert emphasizes the need for exceptional scientists capable of rigorously proving mathematical principles to distinguish between hype and genuine advancements. He remarks, “We urgently need more outstanding scientists who can mathematically demonstrate what is feasible and what is not, enabling us to differentiate between exaggerated claims and substantive progress. MATH+ can significantly contribute by employing mathematical precision to evaluate the efficacy of various approaches.” This contribution, he asserts, “is unique and indispensable.”
Original publication:
Towards provably efficient quantum algorithms for large-scale machine-learning models,Junyu Liu, Minzhao Liu, Jin-Peng Liu, Ziyu Ye, Yunfei Wang, Yuri Alexeev, Jens Eisert & Liang Jiang, Nature Communications volume 15, Article number: 434 (2024), https://www.nature.com/articles/s41467-023-43957-x.
Short bio:
Jens Eisert has been Professor of Theoretical Physics (Quantum Theory) at Freie Universität Berlin since 2011. Before that, he was a professor at the Universität Potsdam and a Humboldt Research Fellow at Imperial College London. In Berlin, he is also affiliated with the Heinrich Hertz Institute and the Helmholtz Center Berlin. He pursued his studies in physics and mathematics in the USA and Freiburg, earning his doctorate in quantum information science from Universität Potsdam in 2001. Eisert is recognized as one of the world’s most cited researchers in the fields of quantum computing and the study of complex quantum systems, contributing significantly since the early days of quantum information science. His research has already garnered three ERC grants. The Berlin newspaper Tagesspiegel listed him among the top 100 researchers of Berlin in October 2023.
LINKS
- Jens Eisert had been or is still involved in the following MATH+ projects:
- AA2-8: Deep Backflow for Accurate Solution of the Electronic Schrödinger Equation
- EF1-11: Quantum Advantages in Machine Learning
- EF1-4: Extracting Dynamical Laws by Deep Neural Networks: A Theoretical Perspective (completed)
- EF1-7: Quantum Machine Learning (completed)
- Jens Eisert at Freie Universität Berlin
- Jens Eisert’s research group at the Dahlem Center for Complex Quantum Systems, Freie Universität Berlin