MATH+ Dissertation Award Winners 2023
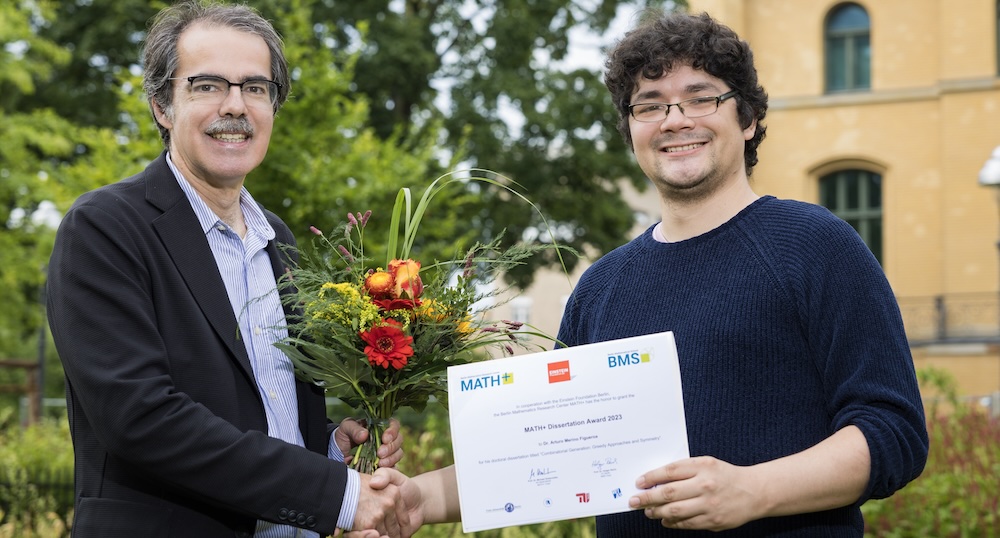
In cooperation with the Einstein Foundation Berlin, the Berlin Mathematical School (BMS) of the Cluster of Excellence MATH+ awards up to three annual prizes for outstanding dissertations to BMS graduates. We are delighted to announce that the MATH+ Dissertation Award 2023 have been presented to Viorel Andrei Bud, Toyomu Matsuda, and Arturo Merino for their excellent theses. Congratulations!
Viorel Andrei Bud
Andrei Bud obtained his Bachelor’s degree in mathematics from Universitatea din Bucuresti (Romania). Prior to his doctoral studies, he completed his Master’s degree in mathematics at Humboldt-Universität zu Berlin. He earned his PhD in mathematics also from HU Berlin as a member of the Berlin Mathematical School (BMS), supervised by Gavril Farkas. His doctoral research focused on the moduli of curves, Hurwitz schemes, Prym varieties, and strata of differentials. Gavril Farkas highlighted his fundamental contributions to a central area of modern mathematics, noting that the dissertation revealed profound new connections between mathematics and physics, showcasing his exceptional talent and impactful work.
Andrei Bud is currently a postdoctoral researcher at Goethe Universität in Frankfurt am Main. As a member of the SFB/CRC 326 “Geometry and Arithmetic of Uniformized Structures,” he works under the guidance of Martin Möller and Martin Ulirsch. His primary research topics include the study of strata of differentials, Prym varieties, and Hurwitz schemes.
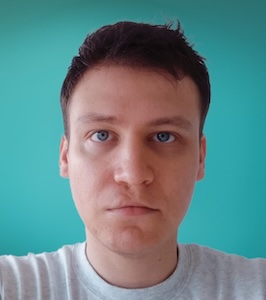
Dissertation Thesis: “Moduli of Prym Curves and Strata of Differentials”
Riemann surfaces are one of the most fundamental objects of algebraic geometry, with important applications in areas such as differential geometry, partial differential equations, or string theory. One crucial aspect in this field is the existence of a very high dimensional parameter space of all Riemann surfaces, and its study has been central in algebraic geometry for more than half a century. In his PhD Thesis, he studied the geometrical classification problem for several parameter spaces of surfaces, such as Prym moduli spaces and strata of differentials. Understanding their geometry provides a meaningful way to study small perturbations of underlying physical objects.
Toyomu Matsuda
Toyomu Matsuda’s academic journey began with a BSc in mathematics from Kobe University (Japan), supervised by Kajino Naotaka, followed by an MSc in mathematics from Kyushu University, under the guidance of Yuzuru Inahama. He earned his PhD in mathematics with the highest distinction, summa cum laude, from Freie Universität Berlin in September 2023, under the supervision of Nicolas Perkowski. During his PhD studies, he was an active member of the International Research Training Group (IRTG 2544 ) “Stochastic Analysis in Interaction” and completed a research stay at the University of Oxford (UK) for half a year, under the mentorship of Rama Cont.
Toyomu Matsuda is currently a postdoctoral researcher at the Swiss Federal Institute of Technology Lausanne (EPFL), where he works within the STOAN group under the direction of Xue-Mei Li. His research interests are deeply rooted in stochastic analysis and stochastic (partial) differential equations, with a particular focus on disordered systems and Gaussian stochastic calculus, including fractional Brownian motion. This robust background and specialized focus underline his significant contributions to the field of mathematics.
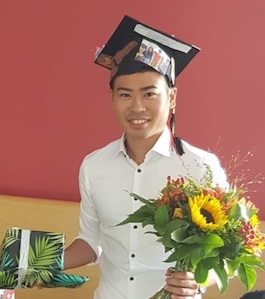
Dissertation: “Fractional Stochastic Calculus via Stochastic Sewing”
Long-range dependence is ubiquitous in empirical studies of data, such as economic time series. As a simple stochastic process exhibiting long-range dependence, fractional Brownian motion was popularized by Mandlbrot. However, due to its long-range dependence, fractional Brownian motion does not fall in the framework of classical probability theory, and it continues to pose many mathematical challenges. The thesis explores new perspectives on fractional Brownian motion, based on a recently discovered tool called the stochastic sewing lemma, which allows us to estimate stochastic Riemann sums. In particular, the thesis introduces its extension, providing sharper stochastic estimates on long-range correlated Riemannian sums. As applications, it proves a highly anticipated conjecture on level crossings of fractional Brownian motion, as well as solution theory of irregular stochastic differential equations driven by fractional Brownian motion.
Arturo Merino
Arturo Merino received his B.Eng. in mathematical engineering and M.Eng. in applied mathematics from Universidad de Chile in 2018, graduating with the highest distinction in both degrees. From 2018 to 2019, he worked as a Project Engineer at the Center for Mathematical Modeling in Santiago, Chile, where he developed heuristics and algorithms for vehicle routing problems. In 2019, Arturo moved to Berlin to pursue his doctoral studies at TU Berlin. He earned his PhD with the highest honors, summa cum laude, in June 2023. His doctoral thesis, supervised by Torsten Mütze und Martin Skutella, was titled “Combinatorial Generation: Greedy Approaches and Symmetry.” Torsten Mütze highlighted Arturo’s exceptional academic and professional achievements, noting his significant contributions to the field of mathematics, particularly in combinatorial generation and algorithm development.
After completing his PhD, Arturo spent a year as a postdoctoral researcher at Universität des Saarlandes and the Max Planck Institute for Informatics in Saarbrücken, Germany, working with Karl Bringmann’s algorithms group. Recently, Arturo started a new position as an assistant professor at the Engineering Institute of Universidad de O’Higgins in Rancagua, Chile.
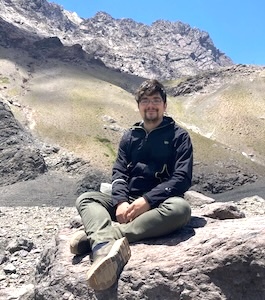
Dissertation: “Combinatorial Generation: Greedy Approaches and Symmetry”
In mathematics and computer science, various combinatorial objects, such as strings, binary trees, and permutations, are frequently encountered. This dissertation focuses on the problem of generating these objects, where an algorithm must visit each object exactly once. The main contributions include efficient algorithms and methods for generating combinatorial objects using Gray codes, as well as an enhanced understanding of the symmetry behind these algorithms.
The first part of this work presents greedy algorithms for generating structured objects. Initially, it addresses elimination trees, which represent possible search paths within a given graph and generalize strings, binary trees, and permutations. Secondly, it develops a new framework for generating objects encoded by binary strings. This method relies on a simple greedy algorithm that computes a Hamiltonian path on the skeleton of any 0/1 polytope. This algorithm can be efficiently implemented if a variant of the classical linear optimization problem is solvable, thus revealing an interesting connection between combinatorial generation and optimization.
The second part of this work explores the concept of symmetry in listings of combinatorial objects. Inspired by Knuth’s conjecture about the middle levels of the hypercube, a new graph parameter called Hamiltonian compression is introduced. This parameter quantifies the symmetry of Hamiltonian cycles in a given graph and provides new insights into an old conjecture by Lovász regarding Hamiltonian cycles in symmetric graphs.