Interview with MATH+ Distinguished Fellow Peter K. Friz (TU Berlin/WIAS)
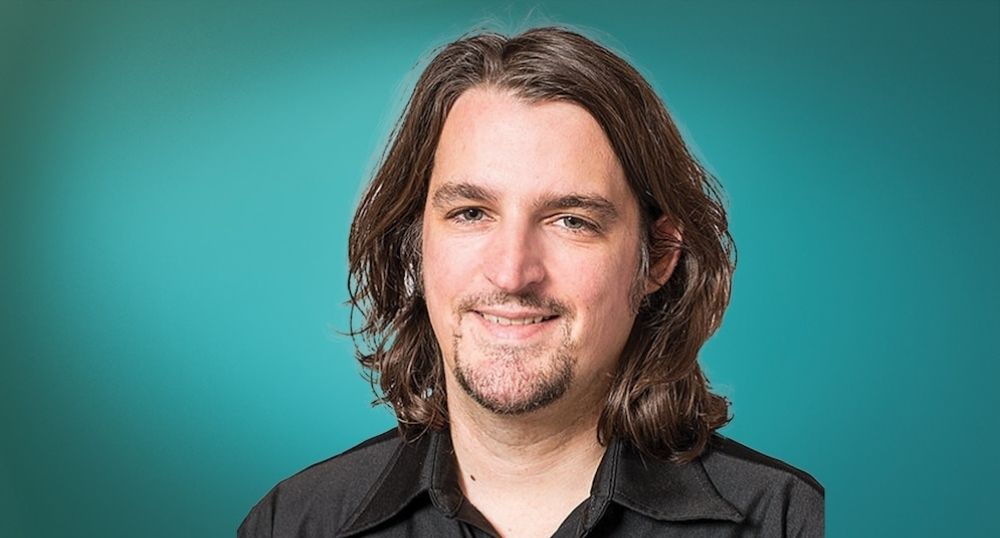
Peter K. Friz, Einstein Professor at Technische Universität Berlin and MATH+ member, has been awarded the MATH+ Distinguished Fellowship for outstanding contributions to the mathematical sciences. In an interview with MATH+, he discusses his academic journey, his research on Rough Path Theory, and future plans, including advancing this field and developing innovative mathematical methods.
Short Bio:
Peter Karl Friz is an Einstein Professor at Technische Universität Berlin (TU Berlin), specializing in stochastic and financial mathematics. He is also affiliated with the Weierstrass Institute for Applied Analysis and Stochastics (WIAS) in Berlin, which, like TU Berlin, is a partner institution of MATH+. His educational background includes studies at the University of Vienna, École Centrale Paris, the University of Cambridge, and New York University’s Courant Institute of Mathematical Sciences, where he completed his PhD under the supervision of S. R. Srinivasa Varadhan in 2004. Before his appointment at TU Berlin and WIAS in 2009, Friz held positions at the University of Cambridge and the Radon Institute in Austria. His research focuses on Stochastic Analysis and Rough Path Theory, areas to which he has made significant contributions. Friz has received prestigious grants, such as an ERC Starting Grant (2010-2016) and an ERC Consolidator Grant (2016-2021), recognizing his impactful work in mathematics. Since 2024, he has been the spokesperson of the Collaborative Research Centre (CRC) SFB/Transregio 388 “Rough Analysis, Stochastic Dynamics, and Related Fields.”
MATH+ Distinguished Fellowship Program
The MATH+ Distinguished Fellowship is awarded to individuals for outstanding contributions to the mathematical sciences. The program aims to support world-leading mathematicians who hold permanent professorships at one of the MATH+ partner institutions, i.e., FU, HU, TU Berlin, WIAS, or ZIB. MATH+ provides Distinguished Fellows with a fellowship allowance to foster innovative ideas and research activities, initiate exciting, vibrant research collaborations, and expand the Fellow’s international research network and activities. There are five MATH+ Distinguished Fellows: Gavril Farkas, Peter K. Friz, Michael Joswig, Bruno Klingler, and Alexander Mielke. For international colleagues, MATH+ offers funding as (Distinguished) Visiting Scholars.
___________________
Interview
After your PhD and research stay in the USA and UK, what brought you to Berlin and attracted you to the positions at TU Berlin and WIAS?
After completing my PhD at the Courant Institute of NYU in 2004, I spent a year on Wall Street before moving to the UK for a tenure-track position at the University of Cambridge. Three years later, I was promoted to associate professor (Reader). During this time, I reconnected with Peter Bank, who had recently moved from New York to TU Berlin, and invited me to Berlin. My family and I found Berlin to be a wonderful place, combining a great city with an excellent mathematical community. I applied for positions at TU Berlin and WIAS and was accepted at both, allowing me to have dual affiliations from the start. A pleasant surprise was that the quality of students in Berlin matched that of Cambridge, which has allowed me to amplify my research in many ways.
What were the most important milestones during your academic career?
To succeed in academia, one needs talent, drive, motivation, and a willingness to work hard. Reflecting on my journey, there is no doubt that one also needs a fair amount of luck to meet the right people who support and believe in you, especially during your graduate student days. But you can help your luck by going to the right place. Personally, I could not have made a better choice than going to the Courant Institute in New York City. Seeing my interests in stochastics, stochastic processes and geometry, my PhD advisor at the time, and later Abel Prize winner, S. R. Srinivasa Varadhan—whose 85th birthday we celebrated this August in Harvard—pointed me most fittingly to the emerging field of Rough Path Theory. His long-term vision and foresight identified it as a future field of greatest importance. Hairer’s rough path solution to the KPZ equation, which earned him the Fields Medal in 2014, was the definitive proof of this; our book has become a reference work in the field. Over all these years, Oxford-based rough path visionary Terry Lyons has been a congenial collaborator and mentor, not only to me but to the entire community, even as it grew larger and—at the price of success—increasingly competitive. We often share our memories of the early days when all “believers” of this new theory could fit into a half-meeting in Oberwolfach!
Could you describe your research in terms that are understandable to the general public or school students?
For example, in school, you learn about smooth functions like cubic or linear functions. You can easily draw these with free online tools like GeoGebra, resulting in smooth and predictable curves and objects. But in the real world, most things are not smooth. Since I also work in financial mathematics, a prime example would be the movements of stock prices, whether it’s the S&P 500 or cryptocurrencies. These prices fluctuate unpredictably, unlike the smooth functions you study at school, and the classical formulas you learn there aren’t useful for modeling such movements.
To handle this, you can use stochastic processes, a concept introduced by arguably the first financial mathematician, Louis Bachelier, in his 1900 doctoral thesis titled “Théorie de la spéculation.” These stochastic processes account for randomness and focus on statistics rather than single outcomes. For instance, in insurance, you average many outcomes to calculate expectations and variances. But in finance, it’s about reducing and managing risks—ultimately, you have to succeed in one specific scenario. Classical calculus, which deals with derivatives and smooth functions, falls short here.
This can be seen as the conceptual motivation for the development of rough path theory, which extends classical calculus to handle such irregularities. It allows you to take some reference noise and build new objects on top of it, like modulations of your noise. Instead of using simple functions as building blocks, rough path theory uses components tailored to the noise in the data. This creates a consistent calculus for differentiating, integrating, and understanding differential equations in this noisy context.
Rough path theory offers a new perspective on classical calculus and complements the classical Itô calculus, a foundational tool in stochastic calculus and one of the great success stories of modern applied mathematics that emerged in the 1950s and ‘60s. Over time, rough paths became invaluable for solving problems that Itô calculus couldn’t handle. Only now are we beginning to truly understand the profound interaction between these two different theories, and it is precisely this interaction that I find most exciting.
BTW, congratulations on your DFG research funding for the Collaborative Research Centre (SFB/Transregio 388) titled “Rough Analysis, Stochastic Dynamics, and Related Fields.“ Can you explain the focus of this research?
“Rough analysis” is quite a recent term; since 2020, it has been included in the important Mathematics Subject Classification (MSC2020). It goes far beyond “Rough Path Theory” and also includes celebrated extensions to rough fields, with groundbreaking work by my colleague and SFB co-speaker, Nicolas Perkowski, at Freie Universität Berlin. It also encompasses important developments like signature theory, which is relevant in data science, with WIAS-based Christer Bayer being a world expert in this area. The core idea of the CRC is to explore and understand the fruitful interaction between rough analysis and stochastic dynamics and how they can enrich each other. Our aim is to develop mathematical methods with potential applications in various fields, including finance, statistics, and data science.
Can you comment on the applications of this research?
While MATH+ is very application-oriented, the work in the new CRC (German: SFB) is more theoretically focused and primarily aims to develop mathematical methods. Nevertheless, we can see numerous synergies. A great example is the emerging field of rough volatility, previously supported within MATH+, which has been immensely successful in the modeling of financial markets.
I am also very excited to have Berlin statistics on board. With the addition of Markus Reiß (HU Berlin) and Vladimir Spokoiny (WIAS), we are joined by leading experts who will help realize rough analysis’ potential in data science and high-dimensional data analysis, especially regarding making robust predictions.
Interestingly, signatures naturally relate naturally to applied algebraic geometry and algebraic statistics, as represented by TU Berlin honorary professor Bernd Sturmfels, MPI director in Leipzig, and Carlos Améndola Cerón (TU). Both are part of our new research centre. Another extremely popular aspect concerns mean field interactions. In a financial context, I explore this together with my co-speaker Ulrich Horst of Humboldt Universität zu Berlin, a renowned specialist in game theory and financial systems.
Lastly, partial differential equations have always been closely linked to applications, and this is no different in the presence of noise. We are well-positioned here, in large part due to our previous DFG research unit, “Rough Path and Stochastic Partial Differential Equations” (2016-2022). I am very excited that Felix Otto (MPI), a distinguished expert on partial differential equations and the most recent recipient of the Cantor Medal, has joined us in this new CRC (SFB/Transregio 388).
Which of the MATH+ projects you have been involved in fascinates you the most?
Let me focus on two recent projects. The first one, within the Emerging Field “Extracting Dynamical Laws from Complex Data,” is titled “Stochastic and Rough Aspects in Deep Neural Networks.” In this project, we successfully used tools from rough analysis to analyze the stability of deep learning networks. Despite their complexity, deep learning networks are mathematically quite simple; they consist of recursions that can be represented on an Excel spreadsheet. Their power lies in handling large dimensions and fitting seemingly chaotic data. One fascinating aspect is the initialization of these networks, which is typically done with Gaussian random variables, aligning with our interest in stochastic processes. By considering deep networks in terms of stochastic differential equations, we were able to provide new stability estimates, making significant theoretical progress. Our findings were published in top journals, highlighting the project’s impact.
Another MATH+ project in the Application Area “Energy Transition” is called “Optimal Control in Energy Markets Using Rough Analysis and Deep Networks.” This project involves applying rough volatility models to energy markets. Rough volatility has proven effective in modeling financial markets, and we found similar scaling behavior in energy markets. This natural combination has yielded the most promising results, demonstrating the practical application of our theoretical work.
How significant is the MATH+ Distinguished Fellow award to you?
Berlin is a fantastic place for mathematics with many brilliant people. I am very honored to have received the MATH+ Distinguished Fellow award. This recognition reflects the trust MATH+ has placed in me, and I am deeply appreciative.
What are your plans related to this recognition and the funding?
I plan to use the funding to extend the collaboration with a valuable postdoc here who is doing some of the most significant work to bring the combination of Itô calculus and rough analysis to a new level. This project, which started two years ago, has inspired scientists in Oslo, Italy, and France to pursue this development. With MATH+’s support, we can continue this important work, further advancing the field and fostering international collaboration.
Thank you very much for the interview and the interesting insights into your research!
(The interview was conducted by Beate Rogler, MATH+.)
LINKS:
- Peter K. Friz’s involvement in MATH+ projects:
- Peter K. Friz’s personal homepage
- Friz at TU Berlin, at WIAS, and at Einstein-Stiftung Berlin
- More about the MATH+ Distinguished Fellowship Program and MATH+ research areas